Self-Learning electrical circuit: A groundbreaking AI discovery
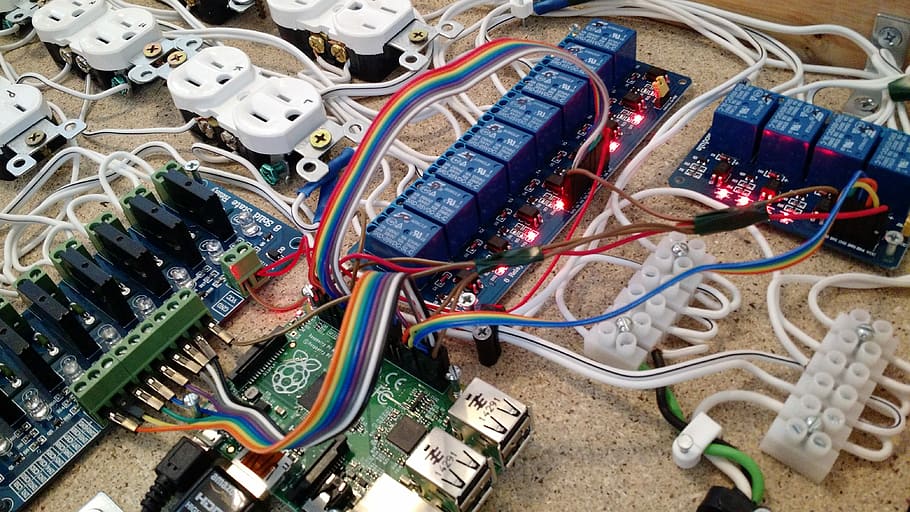
In an exciting development, a simple electrical circuit has displayed remarkable abilities to recognize flowers based on their petal size. Although this may seem like a small feat compared to the advanced AI systems that can recognize faces and transcribe speech, this tiny circuit sets itself apart in a unique way – it learns without any external assistance. Much like a living brain.
Unlike conventional machine learning systems that require massive amounts of computation to fine-tune an AI system, this electrical circuit teaches itself. It offers a potential solution to a significant roadblock in the development of increasingly complex AI programs. The research was presented by physicist Samuel Dillavou at the annual meeting of the American Physical Society held in Chicago. This marks a significant step forward in our understanding of how machines learn.
It’s independent – doesn’t rely on computers
Dillavou emphasizes that the study is a “proof of principle,” highlighting the potential for further research in this field. The findings demonstrates capabilities of electrical circuits previously unknown. They suggest that there may be alternative methods to teach AI systems that do not rely on computers.
The implications of this research are significant for the future of AI. As AI programs grow more complex, the amount of computation required to fine-tune them grows exponentially. However, the ability of this simple electrical circuit to learn independently could pave the way for more efficient and sustainable approaches to developing advanced AI systems.
A Self-Learning Physical System
Artificial neural networks have revolutionized the field of machine learning. But, they come with a significant challenge. They exist only in the digital world, and their training requires extensive computation. This limitation has driven physicists to explore AI electrical circuit that can tune themselves without external computation. This leads to a groundbreaking discovery by Samuel Dillavou and his team that could transform how we teach machines.
Their system uses simple electrical components to tune itself without external help from a computer. The researchers created a network using 16 adjustable resistors, and the system learned to produce the desired output, teaching itself how to perform a given task. What’s remarkable about this system is that it mimics how a living brain learns. It outperformed traditional machine learning systems in its ability to teach itself.
Artificial neural networks rely on connections between nodes, and the optimization problem becomes daunting with the size of the network. Dillavou’s self-taught system could be a solution to this challenge. This model uses physical systems that can efficiently tune themselves, minimizing the amount of computing and memory required to train a such a system. This breakthrough could lead to more complex and efficient AI systems.
Bringing AI Closer to the Future
The potential applications of this discovery are vast. Machines that learn like humans, with the ability to adapt and improve their performance over time, could transform multitude of industries.
“If it’s made out of electrical components, then you should be able to scale it down to a microchip. I think that’s where they’re going with this.”
Jason Rocks, a physicist at Boston University
This breakthrough could pave the way for the creation of more efficient and intelligent machines, bringing us one step closer to the future of AI.
Is this AI electrical circuit different from Traditional Machines?
Unlike traditional machine learning systems, this system mimics how a living brain learns, teaching itself how to perform a given task without requiring vast amounts of computation. Using a network of 16 adjustable resistors, each acting as an edge in the network, the team was able to create a system that was capable of producing desired output by adjusting the resistors.
“It’s a proof of principle. We are learning something about learning.”
According to Samuel Dillavou, the lead researcher on the project.
The system was tested on a variety of simple AI tasks, including the ability to distinguish between three species of iris. Results showed 95% accuracy based on physical measurements of the flower. This is a canonical AI test that traditionally requires a standard catalog of 150 sets of measurements; with 30 used to train the network. It shows this approach may be highly effective.
What’s Next?
While it’s unlikely that the AI electrical circuit network will replace standard neural networks, which can make fine distinctions with great accuracy, the discovery has enormous potential for developing more efficient and intelligent machines. As physicist Jason Rocks notes, “If it’s made out of electrical components, then you should be able to scale it down to a microchip. I think that’s where they’re going with this.”
The ability to create physical systems that can teach themselves has the potential to transform whole industries. This is paving the way for the development of machines that learn as humans do. A significant step forward in the development of more complex and efficient AI systems – with their endless usecases.